Generative AI
BLOG
9 min read
A Comprehensive Guide to Generative AI: Tools, Models, Applications, and Use Cases
Have you wondered how machines can create such realistic images, captivating music, or even write human-quality text? The answer lies in the fascinating world of generative AI. Generative AI is a subfield of artificial intelligence (AI) that focuses on creating new and original content. In this comprehensive blog, you will learn more about how this technology can transform your projects and workflows. Let’s get started!
Need guidance on choosing the right generative AI solution for your business?
Contact Us Today!What is Generative AI?
In simple terms, Generative AI is a type of artificial intelligence that learns patterns from existing data to create fresh and innovative content. Using algorithms like ChatGPT, DALL-E, and BARD, it can generate diverse forms of content such as images, audio, simulations, text, code, and videos.
Generative AI is not just limited to creative applications. It plays a significant role in various industries, helping to automate processes, enhance productivity, and solve complex problems. For example, in healthcare, it can create personalized treatment plans by analyzing patient data. Similarly, in finance, it can generate predictive models for market trends.
Moreover, Generative AI is continually evolving with researchers and developers constantly finding new ways to improve the accuracy and efficiency of generative models, making them more powerful and versatile.
History of Generative AI
The concept of generative AI has been around for decades, but it is only in recent years that we have seen truly amazing advancements in this space. The roots of Generative AI can be tracked back to the 1950s and 60s, when researchers first started exploring ways to get computers to generate new content. These early models were relatively basic, able to produce simple images or short snippets of text.
The real breakthrough came in the 2010s, with the rise of deep learning – a powerful form of machine learning that uses artificial neural networks to learn from vast amounts of data. Deep learning models were able to capture much more complex patterns, paving the way for more advanced Generative AI.
In recent years, we have seen great progress in Generative AI models like GPT-4, DALL-E, Stable Diffusion demonstrating the ability to generate unbelievably realistic and creative content. These models are trained on huge datasets, allowing them to learn the underlying structures and patterns of different types of content. They can then use this knowledge to create brand new, original material that often looks and sounds just like it was made by a human.
Though generative AI models offer endless possibilities, it is equally important to thoughtfully consider their implementations, including where and how they should be used, as well as their ethical implications. These tools are designed to augment human capabilities and simplify tasks.
Want to learn how generative AI can simplify complex business processes?
Talk to Our Experts!How Does Generative AI Work?
There are many different ways that generative AI models can work, but they all share a common goal: to learn the underlying patterns in a dataset and then use those patterns to generate new data. Some generative AI models work by training on a large dataset of images or text, learning patterns to create similar outputs.
Others, like Generative Adversarial Networks (GANs), use a technique called adversarial training where two models are pitted against each other. One model, called the generator, tries to generate new data that is indistinguishable from the real data. The other model, called the discriminator, tries to identify whether the data it is seeing is real or fake. Over time, the generator and discriminator learn from each other, and the generator becomes better at creating realistic data.
GANs and Variational Autoencoders (VAEs) have become highly effective generative models used in text and image generation. After training a model like GAN or VAE, a generative AI algorithm can generate fresh outputs that closely resemble the data it was trained on.
According to a study by OpenAI, GANs and VAEs have shown impressive results in image synthesis tasks, with human evaluators being unable to distinguish between real and generated images in 53% of cases. However, it is important to note that the implementation of generative AI can incur higher costs compared to discriminative AI due to its heightened processing demands.
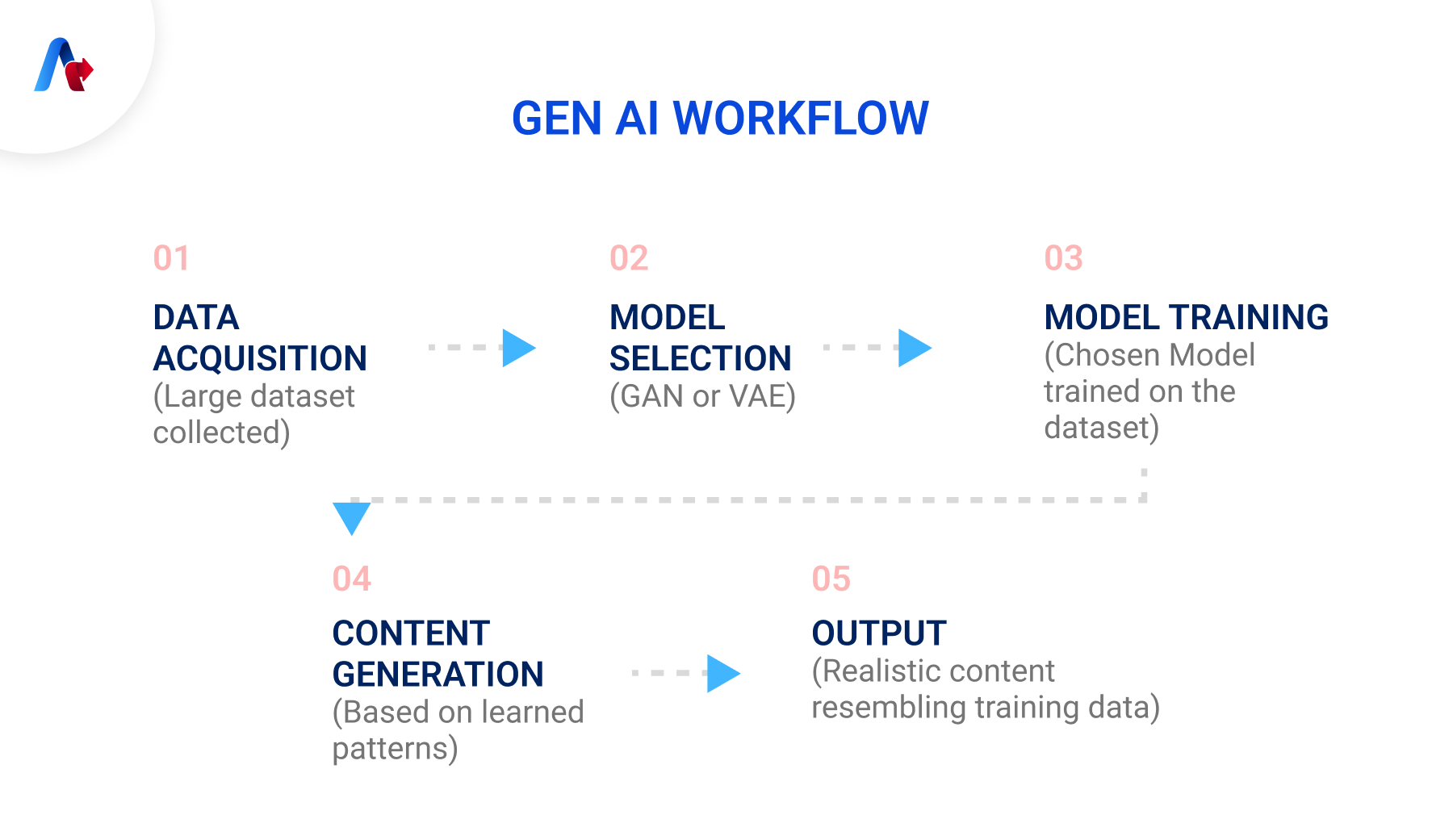
What Are the Benefits and Applications of Generative AI?
Generative AI can help individuals and organizations alike by:
- Personalized Recommendations: Crafting lifelike depictions of individuals (for applications like creating realistic avatars or generating personalized product recommendations).
- Smart Email Responses: Streamlining email responses and optimizing email response efficiency (through tools like smart replies or generating personalized email drafts).
- Tech Support Data: Improving the efficiency of addressing specific technical inquiries (through chatbots trained on technical support data).
- Simplifying Complex Information: Crafting a cohesive storyline by condensing intricate information (useful for journalists or content creators summarizing complex topics).
- Content Creation on Autopilot: Enhancing the content creation process through automation (generating product descriptions, social media posts, or marketing copy).
- AI-powered Research and Innovation: Efficiently analyzing and comprehending pre-existing content while seamlessly generating fresh and original content automatically (summarizing research papers, generating new product ideas, or creating variations of existing marketing materials).
Read More: Transformative Impact of Generative AI on IT Services Organizations
Types of Generative AI Models
There are many different Types of Generative AI Models, each with its own strengths and weaknesses. Here are the TWO most common types:
Generative Adversarial Networks (GANs):
Generative Adversarial Networks or GANs are composed of two sets of neural networks: the generator and the discriminator, which operate in an adversarial way. The generator's primary function is to generate new samples, such as text or images, that closely resemble the training data. On the other hand, the discriminator's main task is to assess the generated data and offer feedback to the generator, and distinguish between the generated samples and genuine ones.
Variational Autoencoders (VAEs):
A Variational Autoencoder (VAE) is a powerful machine learning model designed to compress data by capturing essential features, structural patterns, and interrelationships. It accomplishes this by encoding the data into a compressed representation with fewer dimensions. This compressed representation can then be used by the model to accurately reconstruct the original data through a decoding process.
Interested in a demo to see generative AI in action?
Contact Us Today!What Are Some Examples of Generative AI Tools?
There are a number of generative AI tools available that can be used to create new and original content. Let’s look at a few significant ones:
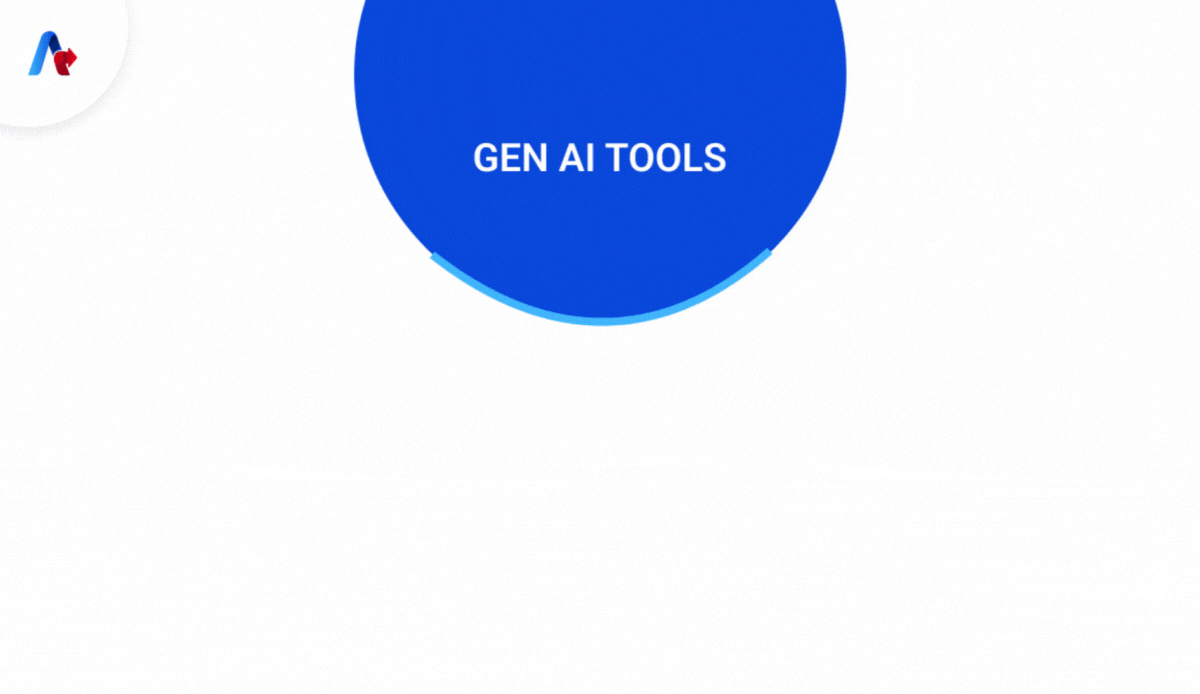
- Writesonic: This multifunctional platform for writing and generating images has gained immense popularity among e-commerce businesses for creating captivating product descriptions.
- DeepDream Generator: An extraordinary open-source platform that unleashes the potential of deep learning algorithms, skilfully creating mesmerizing and otherworldly images that evoke the essence of surrealistic dreams.
- GPT-3 Playground: This platform empowers users to interact with OpenAI's GPT-3 language model to effortlessly generate text through prompt-based interactions.
- GitHub Copilot, Codex: Designed to simplify the process of coding, these code generation platforms use Generative AI to offer a uniquely seamless and error-free coding experience to both professional and amateur developers.
- MuseNet, Amper: These exceptional music generation applications leverage the power of Gen-AI to allow users to create unique and authentic music.
- ChatGPT, Jasper: These Gen-AI-powered chatbots and writing programs are popularly used for authentic and accurate text generation.
- Midjourney, Dall-E: These amazing Generative AI-powered platforms help users generate spectacular image content by describing the image's content.
What Are Use Cases for Generative AI?
Generative AI can transform business experiences if utilized the right way. Here are some examples:
1. Refining Customer Experience:
Chatbots leveraging the power of Generative AI can empower customer engagement by resolving inquiries and personalizing marketing communications.
2. Investment Portfolio Management:
By harnessing the power of Generative AI, investment firms can conduct comprehensive market data analysis and, by leveraging historical performance and current market trends, can generate accurate predictions, optimizing portfolio management and enhancing investment portfolios, ultimately resulting in improved outcomes.
3. Fraud Detection in Finance:
By leveraging an individual's transaction history, Finance can utilize Gen-AI models to enhance its fraud detection systems, enabling more effective monitoring and identification of fraudulent activities.
4. High-Quality Gaming Content Generation:
Generative AI can help gaming companies create and design game content and levels, leading to more engaging and nuanced gaming experiences.
5. Medical Industries:
Generative AI offers the medical industry an efficient means to identify highly promising drug candidates.
6. Data Augmentation:
Gen-AI can be utilized for data augmentation purposes, generating synthetic data to complement limited training datasets, thereby enhancing the performance of machine learning models.
Read More: Future Trends in Generative AI Governance and Security
Best Practices of Generative AI
As with any powerful technology, it's important to use generative AI responsibly. Here are a few best practices to keep in mind:
- Be Transparent About the Use of Generative AI: Let users know when they are interacting with content that was created by generative AI.
- Avoid Bias: Generative AI models can perpetuate biases that exist in the data they are trained on. It's important to be aware of these biases and take steps to mitigate them.
- Use Generative AI for Good: Focus on using generative AI for positive applications that benefit society.
Drive Process Evolution with Generative AI Today!
The remarkable capabilities and user-friendly nature of generative AI models like ChatGPT and Writesonic have displayed immense potential for integrating generative AI into various applications. Simultaneously, advancements in AI development platforms have the potential to greatly accelerate progress and foster innovation in generative AI capabilities across diverse domains, including text, video, images, audio, 3D content, supply chains, logistics, and business processes.
These advancements are touted to shape the future of AI research and development, bringing about transformative and profound changes across the tech landscape. While the new standalone tools are commendable, the true transformative power of generative AI lies in seamlessly integrating these capabilities into our existing toolsets, unlocking even greater possibilities. In short, the present and the future of generative AI is full of potential, but it all depends on how we work with it to achieve greater things.
To explore and experience the true capabilities of generative AI, it is recommended to partner with a trusted Gen AI service provider like Accelirate and enable your team to redefine your processes and achieve desired outcomes.